Harnessing the Power of Bounding Boxes in Data Annotation Tools
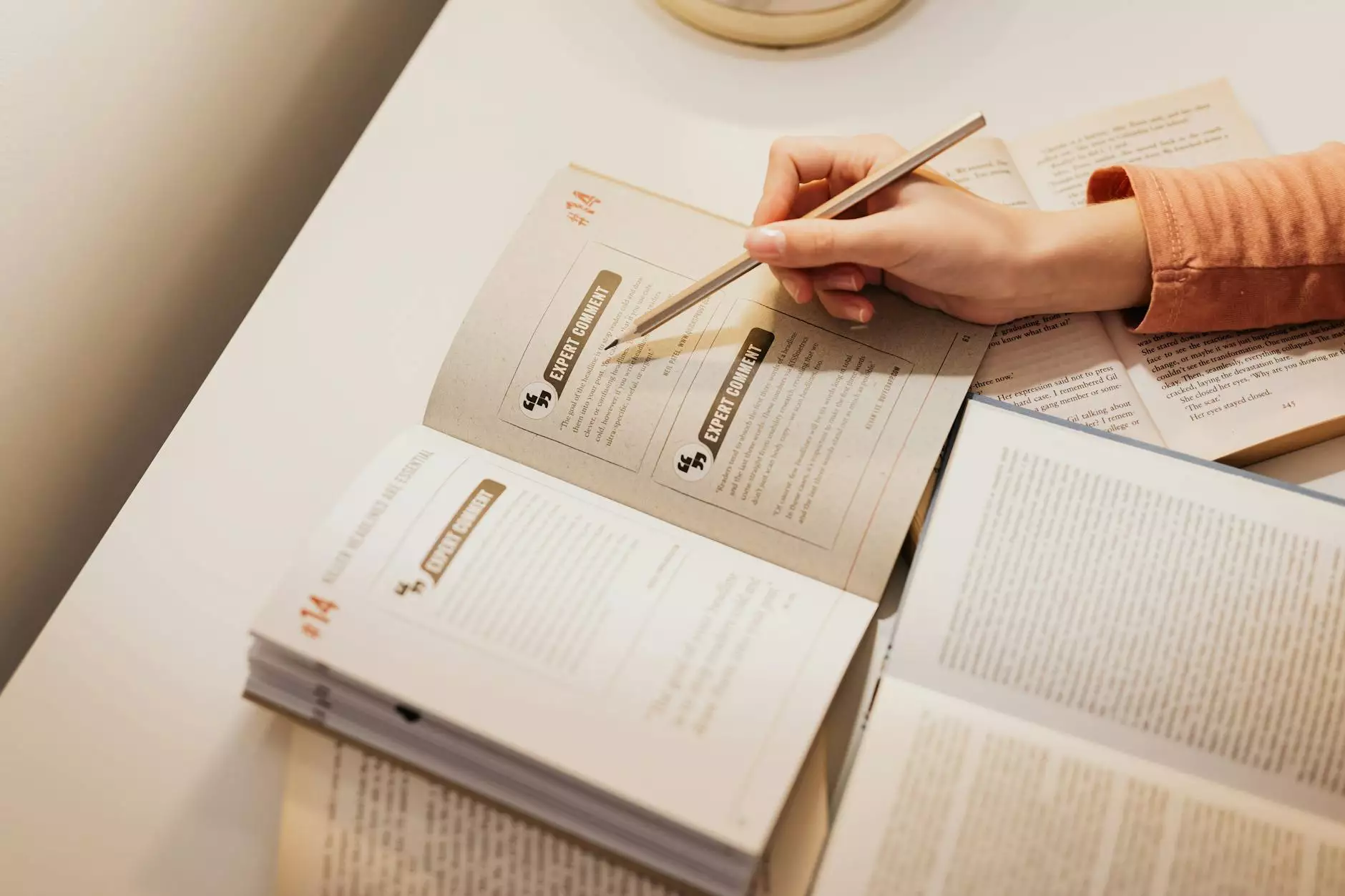
In the ever-evolving landscape of machine learning and computer vision, the importance of precise and efficient data annotation cannot be overstated. The term “bounding box” has emerged as a crucial concept within this field, serving as a foundational element for various applications including image classification, object detection, and more. This article delves deep into the significance of bounding boxes and their integral role in enhancing data annotation platforms like those offered by Keylabs.ai.
Understanding the Bounding Box: A Definition
A bounding box refers to an imaginary rectangle that encapsulates an object within an image. It is defined by the coordinates of its corners, typically the minimum and maximum values along the X and Y axes. This simple yet critical representation allows algorithms to comprehend the spatial dimensions of objects, paving the way for advanced features in computer vision tasks.
Why are Bounding Boxes Important?
Bounding boxes serve multiple pivotal functions in the field of data annotation:
- Object Localization: They help identify the precise location of objects in an image, which is fundamental for machine learning models aimed at object detection.
- Training Data Creation: They are essential for creating labeled datasets that train algorithms to recognize and classify various objects accurately.
- Data Precision: Enhancing the quality of annotated data leads to improved model performance and accuracy.
- Algorithm Efficiency: Properly defined bounding boxes facilitate faster and more efficient training of machine learning models.
The Process of Creating Bounding Boxes
Creating bounding boxes involves several steps, with each step contributing to the overall quality of the data annotation process:
1. Image Acquisition
The first step is obtaining high-quality images. Images can be sourced from various domains depending on the project requirements, such as surveillance footage, sports broadcasts, or satellite imagery.
2. Annotation Tools
Using advanced data annotation tools is crucial for effectively creating bounding boxes. Keylabs.ai offers intuitive platforms that facilitate the quick and accurate annotation of images, allowing users to draw bounding boxes around relevant objects with ease.
3. Quality Assurance
After creating the initial bounding boxes, quality assurance checks are necessary. This stage involves verifying that the bounding boxes accurately encapsulate the intended objects without any significant errors, ensuring the integrity of the dataset.
Best Practices for Annotating with Bounding Boxes
To maximize the effectiveness of bounding boxes in data annotation, following best practices can significantly enhance the quality of your dataset:
- Consistency: Maintain a consistent approach to how bounding boxes are drawn. This includes uniformity in size and shape relative to different objects.
- Clear Guidelines: Provide clear annotation guidelines to all annotators, ensuring everyone adheres to the same standards.
- Use of Tools: Utilize advanced tools that provide automation features, such as auto-completion and adjustment suggestions, which can speed up the annotation process.
- Regular Maintenance: Continuously review and update the bounding boxes to reflect any changes in object shapes or sizes, particularly in dynamic environments.
The Role of Technology in Facilitating Bounding Box Annotation
Technology plays a crucial role in the accuracy and efficiency of creating bounding boxes. Modern data annotation platforms incorporate features that leverage artificial intelligence to enhance the annotation process:
1. Automated Annotation
Many platforms now offer automated annotation features that can automatically suggest bounding boxes based on trained algorithms, significantly reducing the manual effort required.
2. Real-time Collaboration
Collaboration tools allow multiple annotators to work on the same project simultaneously, improving the speed and efficiency of the data annotation process.
3. Advanced Visualization Tools
Visual feedback during the annotation process ensures that annotators can see their work in real-time, helping them adjust bounding boxes as necessary to ensure accuracy.
The Impact of Quality Bounding Boxes on Machine Learning Models
The quality of bounding boxes directly influences the performance of machine learning models. High-quality annotated data leads to:
- Improved Accuracy: Models trained on precise data are more likely to achieve high accuracy rates when identifying objects in unseen images.
- Reduced Overfitting: Well-defined bounding boxes help prevent overfitting, allowing algorithms to generalize better across different scenarios and environments.
- Faster Training Times: Efficiently annotated data can drastically reduce training time, allowing for quicker iterations and deployment of models.
Case Studies: Success Through Bounding Box Annotation
Numerous companies have reaped the rewards of implementing highly efficient bounding box annotation practices:
1. Autonomous Vehicles
Companies like Tesla rely heavily on bounding boxes to train their self-driving algorithms. Accurate annotations enable vehicles to identify pedestrians, other cars, and obstacles in real time, ensuring safer driving experiences.
2. Retail and Inventory Management
Retailers use bounding box annotations in their surveillance systems to monitor stock levels and manage inventory efficiently, automatically detecting when items need to be restocked.
3. Healthcare Imaging
In healthcare, bounding boxes are utilized to annotate medical images, aiding in the diagnosis of conditions through automated image analysis tools, ultimately improving patient outcomes.
Choosing the Right Data Annotation Tool for Bounding Boxes
Selecting a data annotation tool is a critical factor in the success of any project involving bounding boxes. Here are important criteria to consider when choosing a tool:
- User-Friendly Interface: The tool should be intuitive and easy to use, even for those without a technical background.
- Collaboration Features: Look for platforms that allow for team collaboration, enabling multiple users to annotate simultaneously.
- Integration Capabilities: The tool should seamlessly integrate with other systems used for machine learning workflows.
- Support and Resources: Ensure the tool offers ample support and training resources to help users maximize its features.
Conclusion: The Future of Bounding Boxes in Data Annotation
As the fields of computer vision and machine learning continue to expand, the importance of effective bounding box annotation remains crucial. With advancements in technology and data annotation platforms like Keylabs.ai, businesses can streamline the process of creating high-quality annotated datasets. The future of data annotation will undoubtedly involve even smarter tools and methodologies that refine how we utilize bounding boxes, paving the way for innovations across various industries.
Investing in the right data annotation tool can set your business on a trajectory towards success in the data-driven world, enhancing model accuracy, improving efficiency, and unlocking the true potential of your machine learning initiatives. Embrace the power of bounding boxes today, and harness the advancements they bring to your data annotation processes.